Machine Learning (ML) has become a buzzword in the tech industry and beyond, with new applications and techniques emerging daily. The field of ML, a subset of artificial intelligence, involves computers learning from data to improve performance, make predictions, or even make decisions without being explicitly programmed.
This guide provides a foundational understanding of machine learning, illuminating its key concepts, applications, and potential impact on our world. Whether you’re taking your first foray into this fascinating field or seeking to solidify your existing understanding, this resource will serve as your comprehensive guide.
Understanding the Different Types of Machine Learning
Machine learning can be primarily classified into three categories: Supervised learning, Unsupervised learning, and Reinforcement learning. As the name suggests, supervised learning involves training a model on a labeled dataset. This dataset has both the features (input) and the target (output) which the model uses to learn and make predictions.
Unsupervised learning, on the other hand, involves training a model on an unlabeled dataset. Here, the model uncovers hidden patterns and structures within the data on its own, without any guidance. Common applications of unsupervised learning include clustering and dimensionality reduction.
Reinforcement learning is a unique type of machine learning where an agent learns to make decisions by interacting with its environment. The agent learns from its experiences, getting positive or negative reinforcement based on its actions, to maximize some long-term reward.
The Crucial Role of Data in Machine Learning
Data is the cornerstone of machine learning. Without data, machine learning models have nothing to learn from. In a nutshell, the quality and quantity of data available can significantly determine the performance of a machine-learning model. A common saying in the field is “garbage in, garbage out.” This means that if the data inputted into the model is poor quality, the output will be equally poor. Therefore, data preprocessing, which involves cleaning and formatting data, is an essential step in the machine-learning pipeline.
Moreover, data diversity also plays a critical role. If the data used to train a model is not representative of the situations the model might encounter in the real world, it could lead to biased or inaccurate predictions. Therefore, ensuring that the training data is diverse and representative is crucial. A feature store, which is a centralized repository for machine learning features, can also help ensure data diversity by allowing teams to collaborate and share diverse datasets. Not only does this lead to better model performance, but it also promotes transparency and fairness in machine learning.
Application of Machine Learning in Various Industries
Machine learning has found applications in various industries, revolutionizing processes and operations. In healthcare, machine learning models help in disease detection, drug discovery, and personalization of patient care. These models are trained on patient data, including genetic information, to predict disease risk or response to treatment.
In the finance sector, machine learning is used for credit scoring, fraud detection, and algorithmic trading. Here, models are trained on historical financial data to make predictions or identify patterns. In the field of transportation, machine learning has facilitated the development of autonomous vehicles. These vehicles are equipped with machine-learning algorithms that enable them to navigate roads and traffic without human intervention.
The Ethical Considerations of Machine Learning
Machine learning, like any powerful tool, comes with significant ethical considerations. One of the most pressing concerns is the potential for machine learning models to perpetuate or even exacerbate existing biases. This can happen if the data used to train the models is biased. Another ethical issue is privacy. Machine learning often requires large amounts of data, which can include sensitive personal information.
Ensuring this data is collected, stored, and used in a manner that respects privacy is a significant challenge. Lastly, the issue of transparency, or “explainability,” is another ethical consideration. Machine learning models, particularly deep learning models, can be complex and difficult to understand. This lack of transparency can make it difficult to hold these models accountable for their decisions.
The Future of Machine Learning
The future of machine learning looks promising, with advancements in technology and increasing data availability. One area for potential growth is in the field of deep learning, a subset of machine learning that models high-level abstractions in data through the use of multiple layers of artificial neural networks.
Machine learning also has the potential to revolutionize industries even further. For instance, in healthcare, we could see machine learning models predicting disease outbreaks or personalizing medicine to the genetic profile of each individual. The ethical challenges posed by machine learning will also likely become more complex. The field will need to grapple with questions about how to ensure fairness, transparency, and privacy in an increasingly data-driven world.
Getting Started with Machine Learning
Getting started with machine learning requires a basic understanding of programming, preferably in Python, and statistics. Python is widely used in the field due to its simplicity and the availability of machine learning libraries like Scikit-Learn and TensorFlow. Once you have a grasp on the basics, you can dive into more advanced topics like different machine learning algorithms and how to implement them, data preprocessing techniques, and model evaluation metrics.
It’s also important to get hands-on experience. Numerous online platforms provide machine learning datasets that you can use to practice building and training models. Participating in machine learning competitions, like those on Kaggle, can also be a great way to apply what you’ve learned and gain experience.
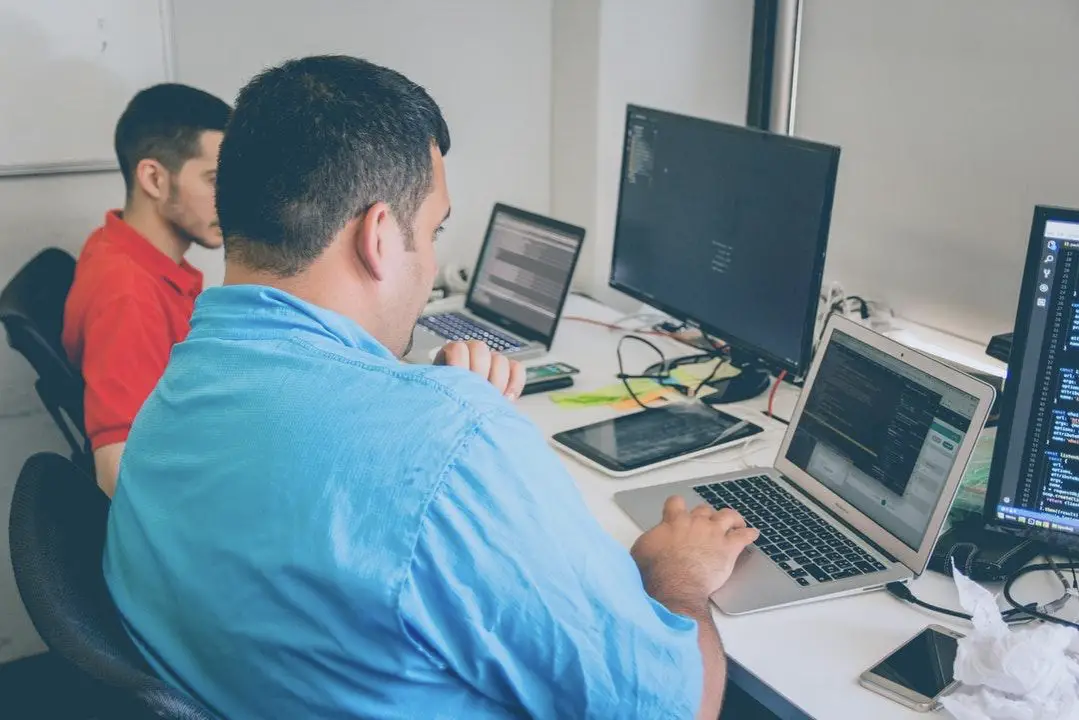
In conclusion, machine learning is a rapidly evolving field with enormous potential to revolutionize various sectors, from healthcare to finance. Its ability to learn from data and improve over time without explicit programming makes it a powerful tool for solving complex problems and making predictions. However, it’s crucial to remember that the effective and ethical application of machine learning depends on high-quality, diverse data and careful consideration of issues like bias, privacy, and transparency.
As we continue to explore the possibilities of this technology, we must also confront its challenges and strive to use it in a manner that benefits all of society. Whether you’re a beginner just venturing into the world of machine learning or an experienced practitioner, continuous learning and hands-on experience are key to mastering this exciting field.